Key Objectives:
AI-Driven Automation: Develop an advanced AI/ML model capable of automatically classifying test questions into relevant medical specialties.
Efficiency Enhancement: Introduce an efficient system that significantly reduces the time and resources required for question classification.
Accuracy Improvement: Ensure superior accuracy in question categorization to uphold the institution’s commitment to high-quality education and assessments.
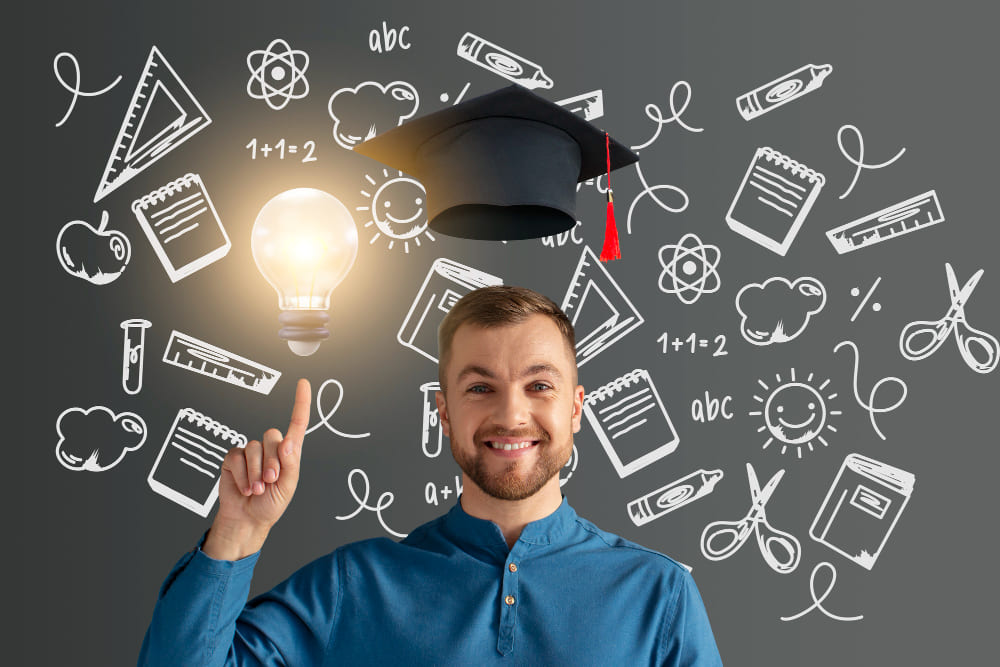
AI/ML Approach:
Our AI/ML experts began by curating a robust labeled dataset of test questions, meticulously categorized by medical professionals into their respective specialties. This dataset formed the foundation for training our cutting-edge AI/ML model. The NLP aspect of our solution involved extracting crucial features and semantic relationships from the textual content of the questions.
Employing state-of-the-art AI/ML techniques, including tokenization, word embeddings, and BiLSTM networks, our model was trained on the labeled dataset. Transfer learning from pre-trained word embeddings enabled our model to grasp intricate patterns in the medical context effectively.
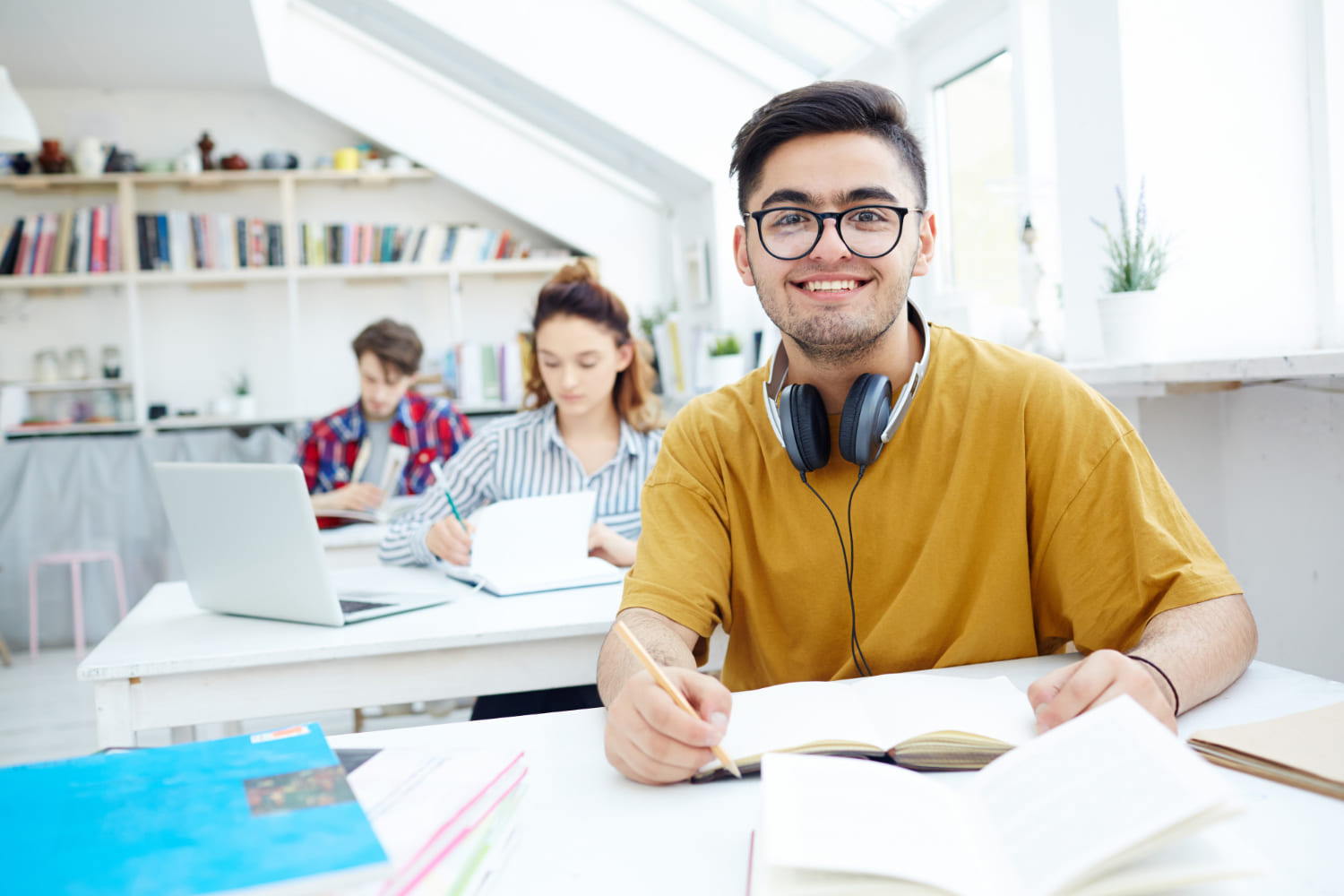
Challenges Addressed:
Limited Labeled Data: Overcoming the challenge of limited labeled data required strategic data augmentation techniques and expert validation.
Class Imbalance: We addressed class imbalance issues by employing advanced sampling methods to ensure fair representation of all medical specialties.
Ambiguous Wording: To tackle ambiguously worded questions, our NLP pipeline integrated contextual information and semantic understanding.
Key Deliverables:
Accuracy Excellence: Our AI/ML model surpassed expectations with an impressive accuracy rate of 92%, outperforming manual classification by medical experts.
Efficiency Gains: Our automated question classification system reduced the time spent on categorization by a remarkable 80%, optimizing faculty members’ productivity.
Consistency and Standardization: Our AI-driven approach introduced consistent and standardized question classification, ensuring fairness and equity in assessments.
Enhanced Learning Experience: By automating question classification, students experienced faster feedback, empowering them to enhance their learning journey.
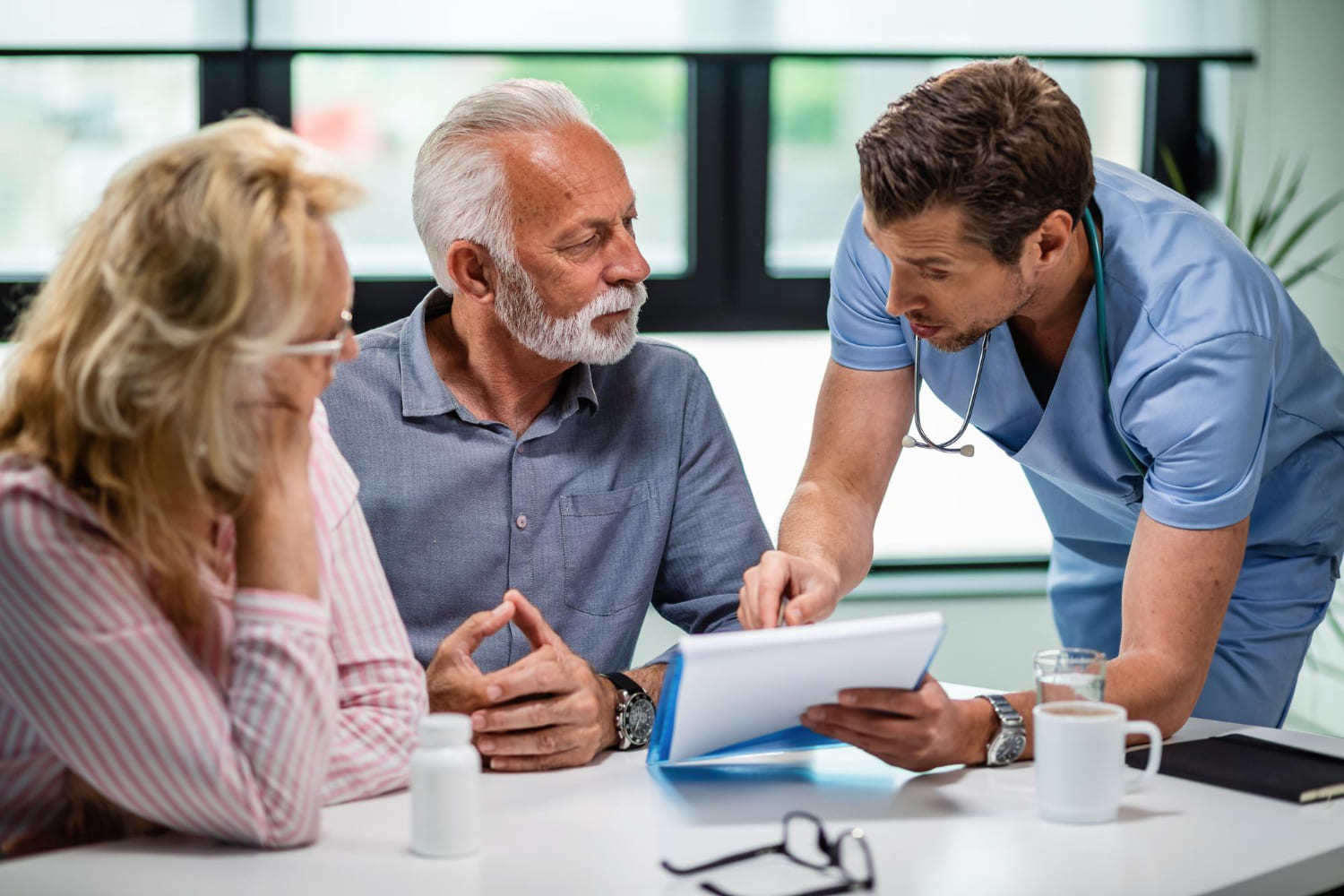
Conclusion:
Through our AI/ML expertise, the medical school achieved a revolutionary transformation in their examination process. Our cutting-edge model delivered unparalleled accuracy, efficiency, and standardization in question classification. As a trusted AI/ML partner, we empowered the institution to prioritize strategic initiatives, foster a collaborative learning environment, and uphold excellence in medical education. Embracing our AI-powered solution, the medical school is now positioned to confidently embrace growth, stay consistent, and provide a world-class learning experience for its students.